You love your customers. They enable your business to, well, be. You want to retain them and worry about which ones might be looking slyly around over their shoulder, wondering if they could have it better with one of your competitors.
Understanding which of your customers is most likely to churn can be an anxious business for a SaaS company. Churn can appear to happen suddenly owing to any number of potential factors, and customers who do churn are unlikely to write you a “Dear John” letter explaining why it wasn’t you; it was them.
There’s a wealth of data on breaking down the likelihood of churn per customer—maybe too much data. When it comes to measuring customer retention rate alone, there are dozens of different metrics that startups use for their retention analysis. What’s more, a simple consultation with Google might suggest to you that the only way to really predict your company’s churn is to build a tailored end-to-end solution of your own.
Nevertheless, there are ways to get a handle on churn prediction, to make the data work for you without having to be an elite-level coder. Making the data work and predicting churn successfully can be the difference between keeping profitable customers and losing them.
What is customer churn prediction?
Customer churn prediction is the practice of analyzing data to detect customers who are likely to cancel their subscriptions. Most of the large subscription businesses conduct their own form of churn prediction analysis to identify customers most at-risk of churning, and, when done well, it leads to huge business savings and improved customer lifetime value (LTV), regardless of size.
Understanding the churn prediction model
For a company to predict churn, historical customer data visualization is combined with machine learning algorithms and logistic regression to rank a customer’s likelihood to churn. Various algorithms are compatible with churn prediction. The machine learning model most associated with this practice is the decision tree model (i.e., Random Forest), which involves the pre-processing of various data sources, followed by training and evaluation.
Companies with their own dedicated data science team can build a customized churn prediction solution that is driven by artificial intelligence. Some prefer this approach given the lack of industry consensus on the exact best way to predict churn—some data scientists prefer survival analysis, or an ensemble model, to the machine learning approach.
If you’re not a coder or your business model doesn’t have the resources or bandwidth to create your own predictive solution, there’s still a variety of predictive analytics services out there, some of which deal with churn rate specifically.
How to leverage churn prediction to prevent churn in the first place
It’s one of the most commonly stated truisms about running a subscription business, but it bears repeating: even seemingly low customer attrition rates can stop businesses from growing or kill them entirely. Even small numbers like 1.0% churn, 2.5% churn, 5.0% churn, are potentially deadly. Let's take a look at a use case below.
Let’s presume that the company in the graph below has a very healthy customer acquisition rate. If it keeps its churn to around 1.0%, its growth (upsells on existing customers and new customers) will be sufficient to keep it out of harm’s way.
If it lets churn go higher than that, it's likely to find it can’t acquire new customers fast enough to maintain its growth trajectory, even if its customer acquisition rate remains the same.
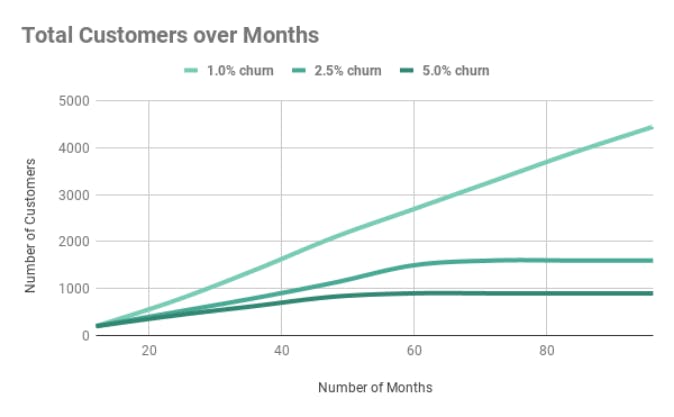
We can see the growth complications that come with higher churn illustrated more fully in the graph below. It’s helpful to think of churn directly in terms of revenue—if your monthly churn is at 5%, that means a revenue loss of 5%. That will compound heavily over time and amount to a huge amount of potential revenue lost by the end of the year.
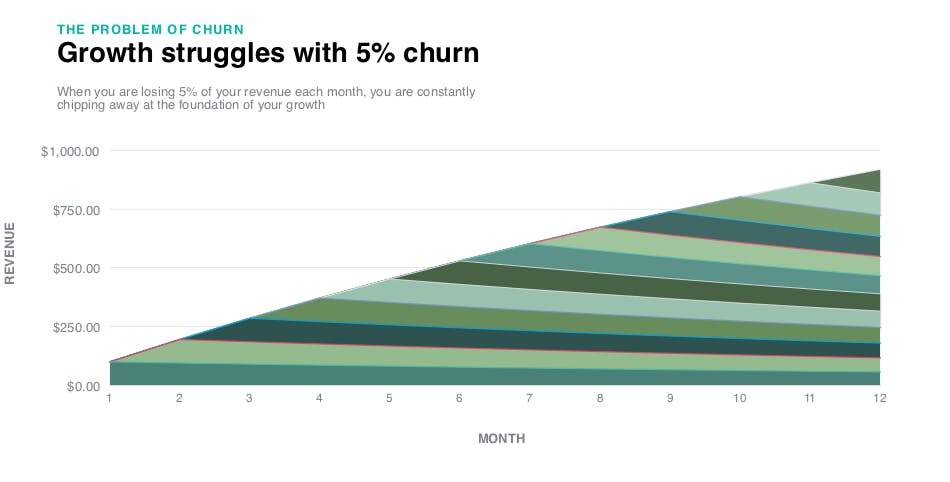
Each band on this chart represents revenue performance for that month. As we can see, each band trends downwards at a greater rate than the last—churn is too high to allow for natural growth.
If you don’t take measures against involuntary churn, you will be endangering the stability of your cash flows, and unstable cash flows mean curtains for a startup.
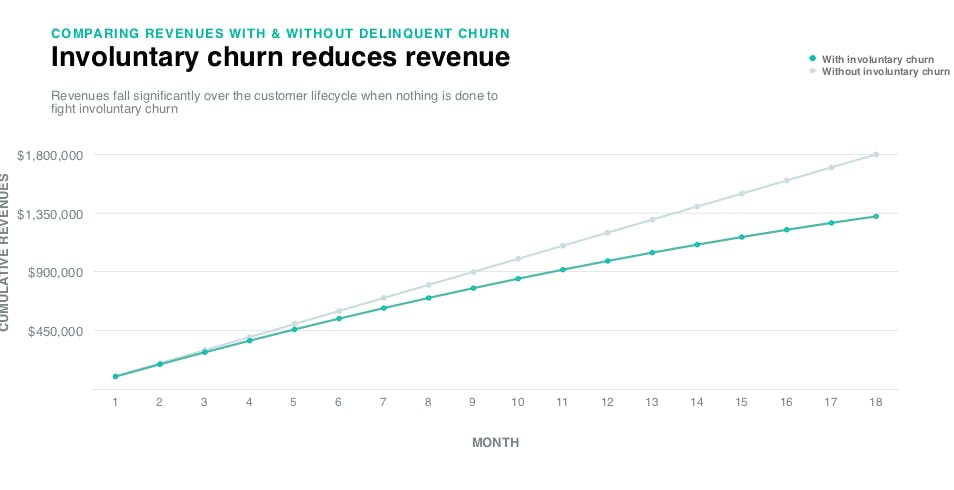
Identify customers with high churn risk
Churn occurs for a variety of reasons. To better understand why a customer has churned, it is key to have proper customer segmentation. A user’s churn probability depends on their overall profile, their customer behavior when using your product, and their needs. A customer’s needs may change over the period of their subscription—not all churn happens in the first few months.
Engage customers before they churn
Harnessed properly, churn prediction can be a major asset in getting a clearer picture of your customers’ experience with your product.
Although the range of potential factors behind churn can be complex, stopping churn often revolves around a tailored approach to improving customer experience. Churn prediction gives you the chance to improve a customer’s experience before they leave for good.
Find business insight in customer churn trends
Churn prediction is not only helpful for preventing imminent churn—it can provide your team with valuable business insights.
On the business development side of things, churn trends can help marketers build customer personas to target a market segment with better messaging and boost customer acquisition.
For retention, your customer success team can anticipate future churn trends and address them proactively to save accounts that would otherwise churn without support.
Let’s take a situation in which a notably large chunk of your customer base churned over a given time frame with no obvious explanation: some were newer users; a few had been using your product for a longer time. Companies oftentimes run into this classification problem: Why did that churn event happen? Some potential reasons could include:
- A poor update
- Exponential growth coupled with high churn at the on-boarding stage
- A new competitor launch that is serving the same product at lower prices
The fact is that all, some, or none of these factors could have prompted the churn—the users may have churned for different individual reasons or for a single interlinked reason. Without an able churn prediction solution and a baseline for data analysis, you won’t be able to connect the dots and recognize which churn trends affect your business most.
The 4 steps to effective churn prediction
1. Reliable customer segmentation
Churn prediction is entirely based around the use of your company’s historical data on your customer. You’ll need your customer analytics to predict how customer churn is affecting your business accurately. Begin by exporting all historical data types that could potentially affect a customer’s likelihood to churn.
These can include:
Demographics and behavioral data
- Is this user a single user or using your product on behalf of their company?
- What is their rate of use of the product in general and the specific features that are available to them?
- How often does this user submit tickets to your support service?
Revenue information for each customer
- Subscription date—is this user a long-term subscriber, or are they new?
- The amount of MRR this customer is individually responsible for— naturally, when it comes to applying your churn prediction, you’ll want to target high-value, at-risk customers first.
Contract terms
- What plan/pricing tier is this customer on?
- How much longer in their plan do they have left pre-expiration? This is a particularly notable piece of data preparation to be able to call on, as delinquent credit card churn is the churn almost any SaaS business will be most susceptible to and one of the hardest to prevent and undo.
Once you have a handle on the historical data you need, divide your customers into segments pertinent to churn prediction, such as the following:
- Customers with multiple upgrades/daily use customers (low risk of churn)
- Customers who communicate regularly (support tickets/calls/upgrade requests) (low risk of churn)
- Customers whose use of the product has declined over the last period (high risk of churn)
- Customers who signed up but did not complete on-boarding (high risk of churn)
- Customers who have never sent in a support ticket/send in many similar support tickets (high risk of churn)
2. Continue with manual data analysis or use a prediction service
Once you have data points, depending on the resources you possess at your company, the predictive model you use to anticipate churn can either come from in-house (a custom solution) or from one of a number of available prediction services.
As we noted above, custom solutions can be adapted to the amount and type of data you wish to analyze, as well as the preferred choice of your data science team where prediction is concerned. If you need to identify complex relationships in your data, then machine learning-based solutions will be the way to go. If you’re looking to answer other kinds of questions, such as looking to predict churn in a certain time period, then survival or hazard functions may be the way to go.
For companies that want to go for a standardized predictive solution, there are a number of different available options, including Paddle’s own solution, Retain, tailored specifically for analyzing, forecasting, and preventing churn.
3. Compare datasets to identify churn causes
Once you’ve fully segmented and analyzed your data, you can see which customers are churners. The correlations you find might include things like:
- High number of customers churning after sign-up due to poorly laid-out pricing tiers leading to customers picking the wrong plan for their needs
- Low levels of communication with long-time customers, leading to a higher degree of delinquent credit card churn as those veteran customers drift away from the product
- Frequent churn spikes following product updates owing to poor visibility/instructive resources
Churn prediction solutions can make these kinds of relationships apparent, allowing you to head off churn with current customers and reduce the likelihood of it happening again. Integrated solutions can also alert you to churn risk in real time—missed payments, for instance, are a sure sign of a customer losing interest in your product and are harder to pick up on single churn prediction reports.
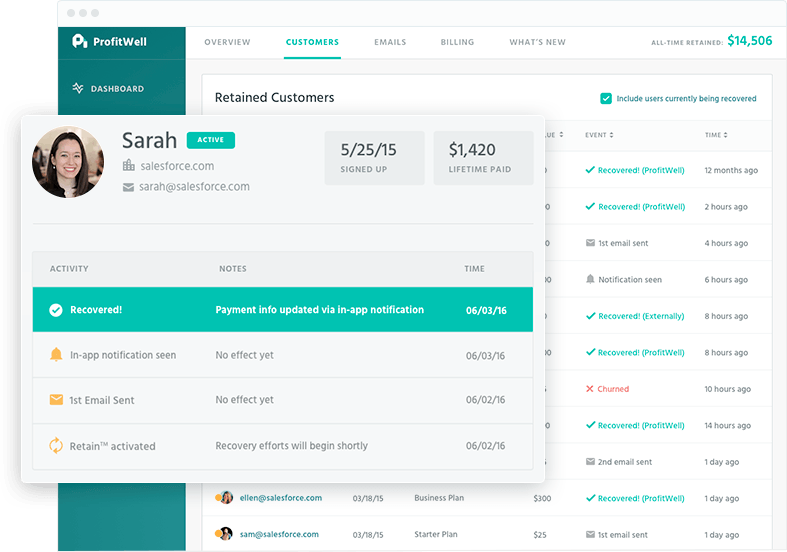
In Paddle's Retain, we can see a customer’s full profile and history of activity. We can manage our outreach and communication with her through the integration, too.
4. Save the customer!
Once you have a sure handle on those customer segments most susceptible to churn, you can begin analyzing what aspect of their relationship with your product is leading to churn-risk. Let’s reuse those examples from above and look at how we could improve the situation:
High number of customers churning after sign-up
Ensure your pricing page is crystal clear regarding what is offered in each plan and which customer types have the most to gain from each one. Stay with customers during on-boarding to make sure any immediate concerns are addressed.
Long-time customer churn
Don’t take the security of veteran customers for granted. Consider incentives specifically targeted to them (discounts, special offers on upgrades, loyalty bonuses) to ensure their eyes don’t wander to your competitors. Maintain a steady communication level, especially in the lead up to contract expiration.
Frequent churn spikes following product updates
Make sure product updates are visible to customers well ahead of time and that changes to functionality are clear. Include resources to help users manage their way around new features or improvements to old ones.
3 common customer churn reasons
Let’s take a look at a few more potential drivers behind customer churn.
Changes in customer circumstance
A customer may find they have gotten all they need out of your product and no longer require your services. In what it says about your quality of service, that’s great: in terms of what it functionally does to your MRR, not so great. Recurring churn of this type may suggest that your range of features is limited.
An account might change hands, and the new account manager may wish to use another software they’re more familiar with, or else a renewal might just get lost between the cracks during handover. This can be a tough one to negotiate but can be improved with dedicated communication.
The state of the competition
A business or customer could find a software they like better than yours and churn to a competitor. This is a particularly damaging form of churn, as your loss is your competition’s gain. This type of churn can relate as much to product performance and pricing as to improving customer service and experience, particularly if a competitor has begun undercutting you on rates. See our article on retention rates by industry to get an idea of how your competitors retain customers.
Changes to product feature/performance
If you’ve changed a feature, removed one, or introduced an unpopular update, a customer may find your product of less use than it once was. Being receptive to your customers’ needs is very important, not only in terms of positioning your product but also how you develop it.
You can find out what your customers really find useful by assessing their use data or just by reaching out to them directly. You ignore this information at your peril—a gung-ho update with a whole roster of feature changes could result in a nasty surprise churn.
How to proactively prevent churn from materializing
Churn prediction can be a battle by itself, so you probably don’t want to hear that it is, in fact, only half the battle. Once you’ve predicted your churn accurately, the next step is to do something to positively affect those customers likely to churn.
1. Communicate value
There’s a whole host of reasons why a customer may be about to churn. One very simple, very common reason: they’re not being helped to get value out of your product. In these cases, communicating with a customer directly can be the best way to turn them from at-risk to satisfied.
Send email nudges to customers not getting the full value from products (i.e., overlooking features) or whose subscriptions are running out, leaving them at risk of delinquent churn. This can be managed easily from a well-optimized CRM or a good churn prediction integration.
2. Incentivize for retention
Promos, discounts, loyalty programs: they all help customers feel valued and wanted. It’s likely to help persuade them to stick around, particularly if your market competition is strong and you’re at risk of being undercut financially or while you’re working on feature updates.
Incentivizing is particularly important in preventing customers who may have unsatisfied needs from churning immediately. Incentives can buy you time while you fix performance issues or expand your service.
3. Analyze churn trends
Not even the best strategy is foolproof. Customers will still churn (though hopefully in hugely reduced numbers) whatever the success of your churn prediction. The key is to resist the urge to bury your head in the sand; look at those churn numbers and find out what went wrong with those customers.
An excellent strategy for churn analysis in general is to plan out your customer’s journey with your product. Once you’ve mapped out the main points, compare the customer’s journey with your churn data, and observe where the risk of churn is highest. Is it during on-boarding? After three months? After two years? After updates?
You can even appeal to customers who’ve churned for direct feedback. They may not feel like providing it, but any feedback of this kind that you can get is extremely valuable.
4. Automate churn reduction
If the whole prospect of putting together entire bespoke churn prediction solutions feels dizzying to you, then an integration aimed at helping you predict and reduce churn is a great fallback option. As we’ve seen, Paddle can command one of the best solutions of this kind on the market, replete with world-class subscription expertise with algorithms that leverage millions of data points to win back customers at exponential rates.
Do business the smart way with churn prediction
Companies often have a scatter-gun approach when it comes to dealing with churn—setting prices as low as possible, gunning for the maximum amount of customer growth possible per month—everything but carefully assessing the factors behind your churn, and making this data work for you. Ultra-aggressive selling techniques might be effective in the short-term, but you're treating the symptoms, not the illness.
A sensible approach to churn prediction—through an integration or your own solution - will allow you to understand the reasons a user might churn and respond to them. A user’s happiness with your product can hinge on the most minor-seeming of conditions. Embracing a predictive solution to handling your churn will give you clarity on each of them, and ultimately help you beat the churn.
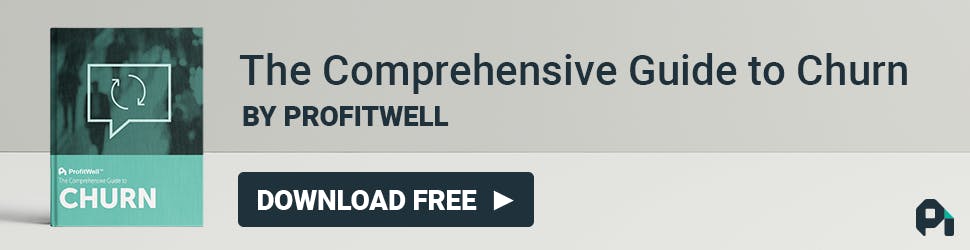
Churn prediction FAQs
What is churn prediction in machine learning?
Machine learning compares new customers to a predetermined “ideal” customer. It uses data-driven algorithms to predict the likelihood of users “sticking around” once they’ve purchased your SaaS platform.
Which model is best for churn prediction?
The machine learning model most associated with churn prediction is the “decision tree model” (i.e., Random Forest), which involves the pre-processing of various data sources, followed by training and evaluation.
What is the difference between churn and attrition?
Customer attrition is another term used to acknowledge the loss of a paying customer or subscriber. “Attrition” is also commonly used to report on employee churn; analyzing the data behind employee turnover and attrition.
What is the difference between a customer churn prediction algorithm and a model?
An algorithm refers to the general approach you will take to predicting customer churn. The churn model is generated once you have executed the algorithm over your training data (and is what you use to inform predictions based on new data).